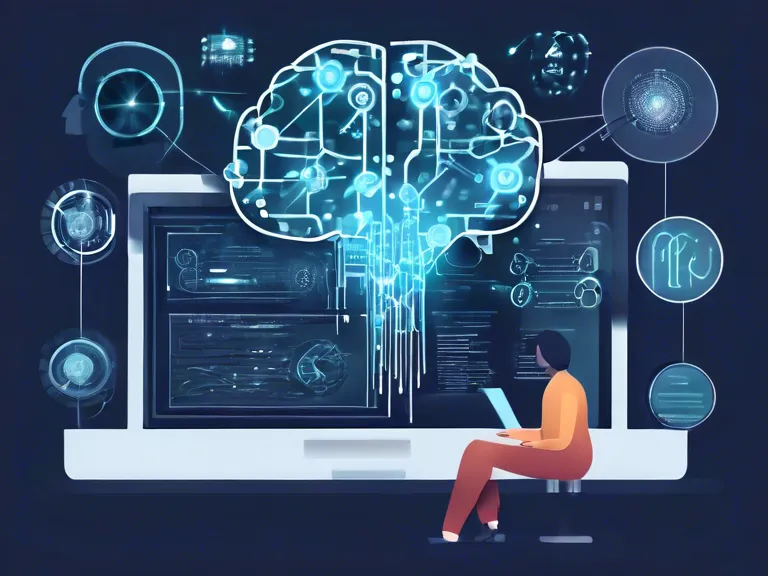
Artificial Intelligence (AI) is becoming increasingly prevalent in our daily lives, from personalized recommendations on Netflix to self-driving cars. However, there has been growing concern over the lack of transparency in AI systems, particularly in the field of Machine Learning. This has led to the rise of Explainable AI, a trend towards making AI models more understandable and interpretable to humans.
Explainable AI is the concept of designing AI algorithms and systems in such a way that their decisions and actions can be easily explained to humans. In traditional Machine Learning models, complex algorithms such as deep neural networks operate as "black boxes," making it difficult to understand the reasoning behind their decisions. This lack of transparency can be a major barrier in critical applications where trust and accountability are essential, such as healthcare, finance, and autonomous vehicles.
The shift towards Explainable AI is driven by the need for increased transparency, accountability, and trust in AI systems. By making AI models explainable, developers and users can better understand how decisions are being made, identify biases or errors, and improve the overall performance and reliability of the system. This trend is also in line with regulatory requirements such as the General Data Protection Regulation (GDPR) in Europe, which mandates the right to explanation for automated decisions.
Techniques for achieving explainability in AI models include feature importance analysis, model interpretability methods, and generating human-readable explanations for decisions. Researchers and practitioners are actively working on developing tools and frameworks that facilitate transparency in AI systems, making it easier for users to understand and trust the decisions made by AI algorithms.
In conclusion, the rise of Explainable AI represents a significant step towards transparency and accountability in Machine Learning. By making AI systems more understandable and interpretable, we can address concerns about bias, errors, and trust in AI applications. As AI continues to play a crucial role in various sectors, the trend towards explainability will be essential in ensuring the responsible and ethical development of AI technologies.